What does a partial regression plot tell you?
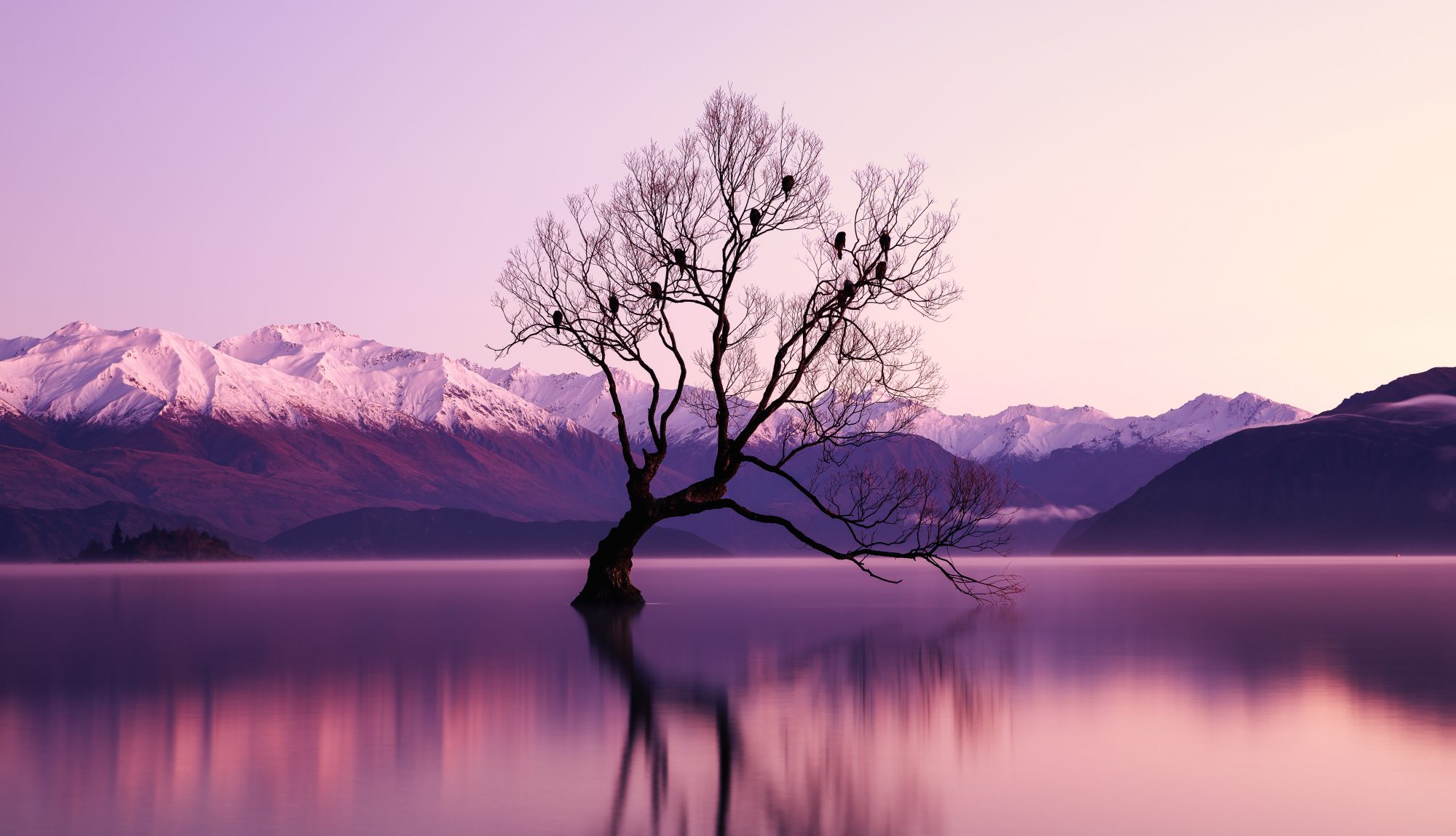
What does a partial regression plot tell you?
Partial regression plots are most commonly used to identify leverage points and influential data points that might not be leverage points. Partial residual plots are most commonly used to identify the nature of the relationship between Y and Xi (given the effect of the other independent variables in the model).
What are the residuals in linear regression?
Residual = actual y value − predicted y value , r i = y i − y i ^ . Having a negative residual means that the predicted value is too high, similarly if you have a positive residual it means that the predicted value was too low. The aim of a regression line is to minimise the sum of residuals.
What does residual in regression mean?
The difference between an observed value of the response variable and the value of the response variable predicted from the regression line.
What is partial regression coefficient?
Partial regression coefficients are the most important parameters of the multiple regression model. They measure the expected change in the dependent variable associated with a one unit change in an independent variable holding the other independent variables constant.
What does the residual plot tell you about the linear model?
The residual plot shows a fairly random pattern – the first residual is positive, the next two are negative, the fourth is positive, and the last residual is negative. This random pattern indicates that a linear model provides a decent fit to the data.
How do you know if your straight enough condition?
The Straight Enough Condition (Assumption of Linearity). The best way to check this condition is to make a scatter plot of your data. If the data looks like it can roughly fit a line, you can perform regression.
Why are residuals important in regression analysis?
Abstract. Residual analysis is a useful class of techniques for the evaluation of the goodness of a fitted model. Checking the underlying assumptions is important since most linear regression estimators require a correctly specified regression function and independent and identically distributed errors to be consistent …
What do residual plots tell us?
A residual plot shows the difference between the observed response and the fitted response values. The ideal residual plot, called the null residual plot, shows a random scatter of points forming an approximately constant width band around the identity line.
What is the meaning of partial regression?
In applied statistics, a partial regression plot attempts to show the effect of adding another variable to a model that already has one or more independent variables. Partial regression plots are also referred to as added variable plots, adjusted variable plots, and individual coefficient plots.
Why do we check the residuals of a linear regression?
Residual plots display the residual values on the y-axis and fitted values, or another variable, on the x-axis. After you fit a regression model, it is crucial to check the residual plots. If your plots display unwanted patterns, you can’t trust the regression coefficients and other numeric results.
How do you tell if a residual plot is a good fit?
Mentor: Well, if the line is a good fit for the data then the residual plot will be random. However, if the line is a bad fit for the data then the plot of the residuals will have a pattern.
Why is it critical that the residuals for a linear regression be normally distributed?
The basic assumption of regression model is normality of residual. If your residuals are not not normal then there may be problem with the model fit,stability and reliability. In order to generalize a regression model beyond the sample, it is necessary to check some of the assumptions of regression residuals.
What does a residual plot for linear regression display?
What is partial regression coefficients?
How do you interpret partial correlation?
How to Interpret the Result. If the partial correlation, r12.3, is smaller than the simple (two-variable) correlation r12, but greater than 0, then variable 3 partly explains the correlation between X and Y.
How do you analyze residuals?
Residual analysis consists of two tests: the whiteness test and the independence test. According to the whiteness test criteria, a good model has the residual autocorrelation function inside the confidence interval of the corresponding estimates, indicating that the residuals are uncorrelated.
What makes a bad residual plot?
Missing higher-order variable terms that explain a non-linear pattern. Missing interaction between terms in your existing model. Missing variables.
What happens if residuals are not normally distributed?
When the residuals are not normally distributed, then the hypothesis that they are a random dataset, takes the value NO. This means that in that case your (regression) model does not explain all trends in the dataset.